Overview
Powered by advanced sensing and Artificial Intelligence (AI) technology, autonomous devices such as self-driving vehicles and UAVs are widely utilized and deployed across the various Internet of Things (IoT) applications. Autonomous devices can be aware of their environment, their state, and incoming data and have the ability to learn and make decisions on their own. For instance, autonomous vehicles and drones can perceive the surroundings, adapt their own behaviors and almost achieve human-level performance in highly complex environment. Autonomous devices and smart appliances, which proactively interface with the IoT world, are establishing a more intelligent and autonomous ecosystem. Even so, intelligence and autonomy raises concerns of performance, privacy, and reliability, and poses demanding challenges on the architecture design and system development for autonomous devices.
ASAD 2019 seeks research on novel architecture, system and algorithms as well as models for autonomous devices to accelerate its development and deployment in the IoT and Autonomy Era. The objective of this workshop is to promote research and development of the computing architecture and system for autonomous devices through dissemination of the state-of-the-art research and discussions between researchers and practitioners. The workshop will be co-located with HPCA 2019.
Call for Papers
The topics to be covered include but are not limited to:
- Innovative architecture and system design for autonomous devices
- Middle-ware and algorithms for performance optimization of autonomous devices
- Run-time scheduling and management for autonomous devices
- Power reduction and energy optimization in autonomous devices
- Security and reliability of autonomous devices
- Network-on-Chip and communication for autonomous devices
- Fault-tolerant architecture and system design and design for testability
- Design tools and optimizations techniques for autonomous devices
The idea behind special focus is general, the workshop will enable discussion and provide a relevant platform among the research community and strive toward maturing research outcome. Any broader topic on Architectures and Systems for Autonomous Devices is welcome.
Schedule
8:45 - 9:00 | Opening Remarks, Yang Hu |
9:00 - 10:00 | Keynote talk: Yanzhi Wang, Northeastern Universty
"A Systematic Framework of Model Compression of Deep Learning Systems for Autonomous Devices" |
10:00 - 10:20 | Andrew Hughes, University of Central Florida
"The Impact of Virtualization on Mixed-Criticality Systems" |
10:20 - 10:40 | Zhendong Wang, The University of Texas at Dallas
"Co-Optimizing Memory Usage and Performance Via Memory Management on Integrated CPU/GPU Architecture" |
10:40 - 11:00 | Nicholas Omusi, University of Central Florida
"Exploration of Cache and Memory Bandwidth Allocation for Real-Time Systems" |
11:00 - 11:20 | Coffee break |
11:20 - 11:40 | Yifan Wang, Wayne State University
"Edge Computing on Connected and Autonomous Vehicles (CAVs)" |
11:40 - 12:00 | Sheng Lin, Northeastern University
"REQ-YOLO: A Resource-Aware, Efficient Quantization Framework for Object Detection on FPGAs" |
Keynote Talk
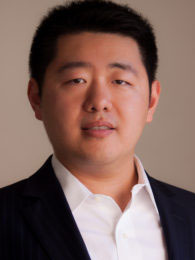
Speaker: Prof. Yanzhi Wang, Northeastern University, USA.
Abstrct: Hardware implementation of deep neural networks (DNNs) with emphasis on performance and energy efficiency has been the focus of extensive ongoing investigations. When large DNNs are mapped to hardware as an inference engine, the resulting hardware suffers from expensive hardware computations and frequent accesses to off-chip DRAM memory, which in turn result in significant performance and energy overheads. We develop ADMM-NN, an algorithm-hardware co-optimization framework for greatly reducing DNN computation and storage requirements by incorporating Alternating Direction Method of Multipliers (ADMM). We can achieve 246X, 32X, 34X, and 17X weight reduction on LeNet-5, AlexNet, VGGNet, and ResNet-50, respectively, with (almost) no accuracy loss. We achieve a maximum of 1,910X weight data storage reduction when combining weight pruning and weight quantization, without accuracy loss. The proposed ADMM-based framework has been applied to the perception and control of UAVs (unmanned aerial vehicles). The object detection and perception of UAV using the YOLO DNN model achieve the currently highest performance and energy efficiency compared with prior work.
Bio: Dr. Wang is currently an assistant professor in the Department of Electrical and Computer Engineering at Northeastern University. He has received his Ph.D. Degree in Computer Engineering from University of Southern California (USC) in 2014, and his B.S. Degree with Distinction in Electronic Engineering from Tsinghua University in 2009. Dr. Wang's current research interests are the energy-efficient and high-performance implementations of deep learning and artificial intelligence systems, as well as the integration of security protection in deep learning systems. His works have been published in top venues in conferences and journals (e.g. ASPLOS, MICRO, HPCA, ISSCC, AAAI, ICML, ICLR, ECCV, ACM MM, CCS, VLDB, FPGA, DAC, ICCAD, DATE, LCTES, INFOCOM, ICDCS, Nature SP, etc.), and have been cited for around 4,000 times according to Google Scholar. He has received four Best Paper Awards, has another seven Best Paper Nominations and two Popular Papers in IEEE TCAD. His group is sponsored by the NSF, DARPA, IARPA, AFRL/AFOSR, and industry sources.
Committee
General Chair:
- Yang Hu, University of Texas at Dallas
Steering Committee:
- Weisong Shi, Wayne State University
- Tao Li, University of Florida
Program Committee:
- TBD
Registration
Please register from the entrance of the HPCA 2019 main conference page , and look over the related program and schedule. Early registration is really recommendation, and applying for HPCA student travel grants is also a good alternative. If you have any questions, please contact general chairs without hesitation.